Internet of Things (IOT)
The possibilities of predictive maintenance
22/10/2021 | Written by: Ronald Teijken and Damiaan Zwietering
Categorized: Internet of Things (IOT)
Share this post:
Wouldn’t it be nice if you could predict more accurately when an asset will need maintenance? IBM has expanded his Enterprise Asset Management platform Maximo Application Suite (MAS) with functionality to support customers in their ´Journey to Predict´. How does this new functionality work and what can you do with it?
Predictive maintenance gives a prediction about an asset: when will it most probably fail and when does which part of it need maintenance? In this article Damiaan Zwietering, IBM Developer Advocate and Datascientist, describes a predictive maintenance approach and the opportunities this approach offers for various assets. Because an asset can be anything, from a production line to a windmill and even a city or patient. Ronald Teijken, Business Partner Manager Benelux for IBM MAS, tells how predictive maintenance in IBM MAS works and what the added value of the new functionality as an integral part of a comprehensive application suite like IBM MAS is.
It’s all about optimization
“Predictive maintenance is about optimization. First you must ask yourself: what do I want to optimize?”, Damiaan Zwietering says. “With traditional asset management an asset is regularly inspected, even when this is not really necessary. That’s inefficient. Another option is to use a ‘run to failure’ method; you just wait until an asset fails. But when it does, integrated processes can also fail, and that makes this approach expensive. That’s why predictive maintenance usually optimizes the uptime. But you can also choose to optimize the maintenance costs or output.
” The more data you use, the better predictive maintenance gets. Zwietering: “After a while you will gather more and more asset data and your predictive maintenance solution will calculate an expected lifetime. “But then one machine suddenly fails sooner than expected and another machine much later. What has caused this difference? Subsequently you start looking for differences between the assets. Maybe one of them is situated in a cooler environment or has another maintenance engineer servicing it?” By using more and more data about various aspects which impact the performance, predictive maintenance will become better and better. “You can push different buttons, measure the outcomes and optimize maintenance based on the results.”
Extra data
In most cases predictive maintenance revolves around the asset itself: how old is the asset, how often has it been used and when was the last time maintenance took place? “But there are much more aspects which impact the lifespan of an asset, like temperature or humidity”, Ronald Teijken says. IBM MAS can assess all possible aspects in its predictive maintenance. “So instead of only measuring if – for instance – a machine is switched on or off, you can now base your maintenance decision on extra data like information from a thermometer or sensor which measures vibrations, but also external data like the weather forecast. This way you can build predictions on a more complete dataset, and you’ll get a more accurate outcome which you can interpret better than before.”
“The predictive maintenance functionality in IBM MAS is part of a comprehensive application suite. That means you can base maintenance decisions on an enormous amount of data coming from all IBM MAS applications”, Teijken explains. All this data is available through a central platform. “You can apply predictive maintenance on all interconnected MAS applications and paired working processes, systems and devices.” On top of that IBM MAS offers an outstanding performance and AI functionality which leads to even faster, more insightful analysis of predictive maintenance data. Deep learning functionality creates a self-learning solution and leads to continuously optimization of asset management.
Capturing knowledge
“It is wise to build and expand predictive maintenance step by step”, Zwietering advises. “When you import all data at once, you will probably create a complex model. With hundreds of parameters which all influence each other. It is impossible for engineers to understand such a model. So there is a big possibility they will not use it in daily practice.” The gradual process of adding parameters has another advantage to it. “When an experienced engineer is building a predictive maintenance solution this way, he will also preserve his knowledge within your organization. For example, he knows that a certain sound indicates a specific machine part is ready for replacement. Using a microphone as sensor and AI for analyzing the sound, you can capture his experience forever. So if he’ll leave your organization, his knowledge will remain.” The predictive maintenance solution in IBM MAS is self-learning. “This way you can create a feedback loop. Experienced engineers formerly had to conduct this loop themselves. What if an engineer, for instance, replaces a sprocket which in an earlier case has caused a failure, and nevertheless the machine fails? Then IBM MAS will log this and will embed this information in his future maintenance advise. This is another way in which knowledge and experience is captured.
Predictive maintenance is applicable on various assets. “You can consider almost everything an asset: a bridge, company footprint, patient, the air pollution in a city or the Covid-situation in a country. Zwietering has been gathering Covid-data from the first Covid outbreak on and is analyzing this in a predictive model he has built. In this model the people of a specific country are the asset. In our next blog about predictive maintenance we will elaborate further on this subject.
Do you want to know more about the the journey to predict?
Tune in the live panel discussion with Waternet and Damen Shipyards at Think Summit 2021, November 4 >

Business Partner Manager Netherlands @ IBM for Asset management, Internet of Things, Supply Chain and AI.
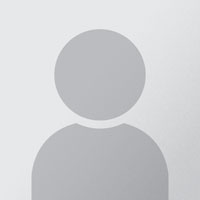
Sales Engineer Data & AI @ IBM
Automate work and accelerate business growth
Many companies need help to navigate the rapid changes that define today’s business environment. To improve their responsiveness and flexibility, they are looking for new ways of conducting business, rethinking their processes, and investing in digital transformation projects to increase the robustness of their operations. They rely on business automation technologies to cut out repetitive […]
Sustainability and the technologies enabling the transition
Creating a sustainable future demands significant technological innovation to decarbonize society, restore biodiversity and ecosystem health, foster thriving oceans for sustenance and economic growth, remove atmospheric carbon, transition to sustainable agriculture, and advance eco-friendly cities that align with our vision for a better future. Generative AI has achieved much in recent years and now surpasses […]
Data-driven asset management with IBM Maximo Application Suite and Cloud Pak for Data
IBM has enhanced its Enterprise Asset Management platform, IBM Maximo Application Suite (MAS), with IBM Cloud Pak for Data: a supporting platform which provides a framework for combining a variety of data from different areas of an organization. How does IBM Cloud Pak for Data help organizations gain additional asset management insights from available data? […]